Artificial Intelligence (AI) is transforming various industries, and one of the most significant changes can be observed in the domain of fraud detection within the realm of credit cards. With the increasing complexity of fraudulent activities, traditional methods often fall short. However, AI has emerged as a potent weapon in combating fraudulent transactions and safeguarding consumer information.
Anúncios
In this blog post, we delve into the impact that AI is having on credit card fraud detection in the United States. We’ll explore the intricacies of this technology, its benefits, and the challenges it faces in implementation. By the end, you’ll have a comprehensive understanding of how AI is reshaping the landscape of fraud prevention.
Understanding AI in fraud detection
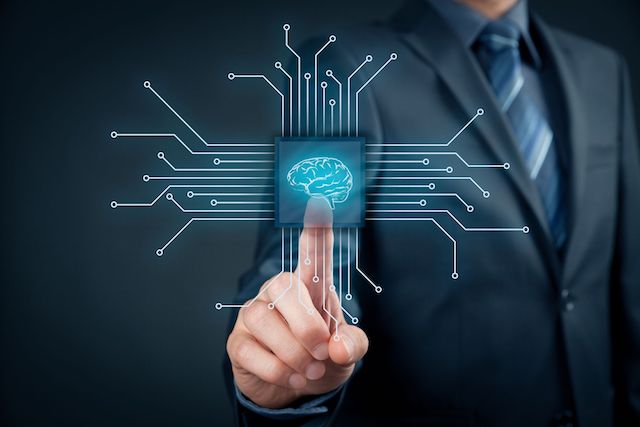
Before delving into how AI is making a difference in the detection of fraudulent credit card activities, it’s essential to understand the basic mechanisms of AI in this context. AI, particularly machine learning (ML), relies on algorithms that are trained to identify patterns and anomalies within large datasets. This ability makes it particularly effective in identifying suspicious transactions that may indicate fraud.
Anúncios
Traditional systems often use rule-based algorithms to detect fraud, which are designed based on predefined rules and patterns. In contrast, AI systems learn from data, making them more flexible and capable of adapting to new types of fraudulent behavior. This adaptability is crucial as fraudsters continuously evolve their methods.
The most common techniques used in AI for detecting fraudulent transactions include supervised learning, unsupervised learning, and deep learning. These techniques can efficiently sift through vast amounts of transactions to identify those that deviate from normal behavior, flagging them for further investigation.
Anúncios
Supervised learning
Supervised learning is one of the primary methods used in AI-driven fraud detection. It involves training algorithms on a labeled dataset where the outcomes are known, categorizing transactions as either fraudulent or legitimate. By learning from historical data, these algorithms become proficient in identifying patterns associated with fraud.
Once trained, the model can apply what it has learned to new transactions, predicting the likelihood of fraud. These predictions enable financial institutions to take immediate action, such as blocking transactions or requesting additional verification from the cardholder, thereby reducing the risk of further unauthorized activities.
Unsupervised learning
Unlike supervised learning, unsupervised learning does not rely on labeled data. Instead, it identifies patterns and anomalies without prior knowledge of what constitutes fraud. This method is particularly useful for discovering new and unknown types of fraud, as it can reveal insights that supervised approaches might miss.
In the context of credit card transactions, unsupervised learning algorithms analyze transaction data to create clusters of normal behavior. Transactions that deviate significantly from these clusters are flagged as potentially fraudulent. This method can detect abnormal patterns that were previously unseen by security systems.
Real-world applications of AI in credit card fraud detection
The practical applications of AI in combating fraudulent activities involving credit cards are vast. Financial institutions, payment processors, and credit card companies have integrated AI algorithms into their fraud detection systems, resulting in a significant reduction in fraudulent activities and associated losses.
One notable application is the real-time monitoring of transactions. AI systems can analyze each transaction as it occurs, assessing its risk based on various parameters such as transaction location, amount, and cardholder behavior. This real-time analysis enables immediate responses to suspicious activities, thereby minimizing potential damage.
Real-time transaction monitoring
The advent of AI has enabled the implementation of real-time transaction monitoring, which is crucial for swiftly identifying and mitigating fraudulent activities. These AI systems can instantly evaluate the risk level of each transaction by considering multiple factors, such as the cardholder’s spending habits, geographical location, and recent activity.
If a transaction appears suspicious, the system can take immediate actions, such as sending alerts to the cardholder, temporarily blocking the transaction, or initiating additional verification procedures. This prompt response reduces the window of opportunity for fraudsters and protects consumer funds more effectively.
Real-time monitoring is particularly valuable for detecting new and emerging fraud schemes. As AI systems continuously learn and adapt to new data, they become more adept at identifying unusual patterns that may signal a fraudulent transaction, thereby providing a robust defense against evolving threats.
Enhanced customer authentication
AI has significantly improved customer authentication methods, making it more challenging for fraudsters to compromise credit card accounts. Traditional authentication methods, such as passwords or PINs, have vulnerabilities that skilled criminals can exploit. AI-driven techniques, however, offer a higher level of security.
For instance, biometric authentication methods such as fingerprint scanning, facial recognition, and voice recognition leverage AI to verify users’ identities accurately. These methods are difficult to replicate, providing a more secure barrier against unauthorized access. Additionally, AI can analyze behavioral patterns, such as typing speed and mouse movements, to authenticate users based on their unique behaviors.
By incorporating these advanced authentication techniques, financial institutions can significantly decrease the likelihood of fraudulent activities. Customers benefit from enhanced security, resulting in increased confidence in their financial transactions and reduced stress over potential fraud.